
Risk and Resilience Management
LAM Laboratory
Associate Professor:LAM Chi Yung
E-mail:
[Research areas]
Risk and Disaster Management, Critical Infrastructure Protection
[Keywords]
Risk, Disaster, Resilience, Infrastructure, Analysis, Simulation
Skills and background we are looking for in prospective students
We welcome students with a good class degree in engineering or science, and interest/experience in numerical and data analysis. Good written and oral communication skills in English are also required.
What you can expect to learn in this laboratory
Our research concerns with the management, communication, and regulation methods for various types of risks in nature and society. In our laboratory, students are expected to understand the theories of risk and risk management processes, and the integrated analytical techniques for handling complex problems relating to risks and disasters.
【Job category of graduates】 Civil servant, consultant company, railway/ transportation/ engineering company
Research outline
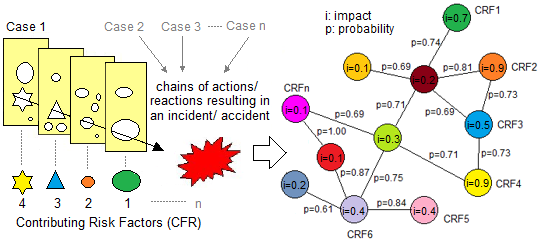
Fig. 1. Sets of incident chains and their transformed interdependent network (with probabilities and impacts).
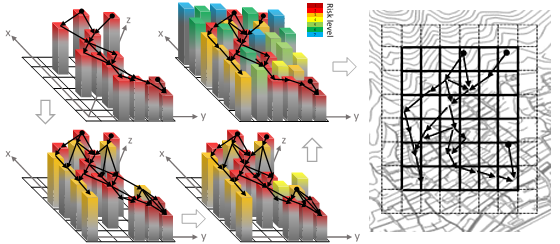
Fig. 2. Predicted time-series changes of risk level and the cascading pathways of disastrous effects in areas.
We are conducting research on modeling, analysis, and prediction of risk cascades and resilience in infrastructure and socioeconomic systems, including (but not limited to):
Risk Cascade Analysis and Prediction
We have applied statistical modeling to quantitatively identify the effects of risks and their cascading effects in infrastructure systems and socioeconomic systems. Network modeling and analysis approaches are also adopted to capture the nonlinear and multiple interdependencies among various disaster risks, so as to reveal the topological complexity for disaster prevention, mitigation, preparedness, response, and recovery (Fig 1).
Risk of Natural Hazards to Infrastructure
We have applied probabilistic models to quantitatively identify the effects and impacts of various risk factors from natural hazards and its cascading effects in infrastructure systems. Different natural hazard scenarios and infrastructure information are considered, and the infrastructure status during natural hazards are then analyzed, so that the expected impacts to a given infrastructure in various extreme levels of natural hazards can be predicted.
Resilience to Risks and Disasters
Resilience refers to the ability to recover from the adverse effects. We have proposed integrated methods to model the impacts of disaster risks, and to develop evidence-based decision-making support systems for societies with greater resilience to disaster risks. This research area explains the observed associations and sequences of change mechanisms between disaster risks and their effects (Fig 2).
Key publications
- LAM, C.Y., et al. (2021). Topological network and GIS approach to modeling earthquake risk of infrastructure systems: A case study in Japan. Applied Geography, 127.
- LAM, C.Y., et al. (2020). Network topological approach to modeling accident causations and characteristics: analysis of railway incidents in Japan. Reliability Engineering & System Safety, 193.
- LAM, C.Y., et al. (2019). Risk analysis for consumer-level utility gas and liquefied petroleum gas incidents using probabilistic network modeling: A case study of gas incidents in Japan. Reliability Engineering & System Safety, 185.
Equipment
Advanced Analytical Software and Analyzers, High Performance Computers, Smart Glasses.
Teaching policy
Our laboratory encourages students to conduct advanced research, and we stimulate students to have creative thinking in the research processes.