Mr. MAI received Best Student Paper Award in ICISSP 2025
Mr. MAI, Trong Khang (3rd-year doctoral student in Beuran Lab, Next-Generation Digital Infrastructure Research Area) received Best Student Paper Award in the 11th International Conference on Information Systems Security and Privacy (ICISSP 2025).
ICISSP 2025 is an international conference, which was held in Porto, Portugal from Feb. 20 - 22, 2025. The Best Student Paper Award is conferred to the author (who is a student) of a paper presented at the ICISSP 2025 conference, selected by the Program/Conference Chairs. The decision criterion considers both the paper quality (assessed by the Program Committee), and the oral presentation quality (feedback given by session chairs at the conference venue).
*Reference : ICISSP 2026 (Previous Awards)
■Date Awarded
February 22, 2025
■Title
CyLLM-DAP: Cybersecurity Domain-Adaptive Pre-Training Framework of Large Language Models
■Authors
Khang Mai, Razvan Florin Beuran, Naoya Inoue
■Abstract
Recently, powerful open-source models LLMs, such as Llama 3, have become alternatives to commercial ones, especially in sensitive or regulated industries. In cybersecurity, most LLM utilization relies on custom fine-tuning or post-training methods, such as prompt engineering. Although domain-adaptive pre-training has been proven to improve the model's performance in the specialized domain, it is less used in cybersecurity due to the cumbersome implementation effort. This paper introduces CyLLM-DAP, a framework for expediting the domain specialization process of LLMs in cybersecurity by simplifying data collecting, preprocessing, and pre-training stages in low-resource settings.
In this paper, we demonstrate how CyLLM-DAP can be utilized to collect, process data, and develop cybersecurity-specific LLMs (CyLLMs) based on state-of-the-art open-source models (Llama 3 and Mistral v0.3). The effectiveness of domain-adaptive pre-training is confirmed via two experiments for text classification and Q&A tasks. Our evaluation results show that, when compared with general base or instruct models, injecting the LLMs with cybersecurity knowledge allows the models to generally perform better in every fine-tuning epoch for the text classification task; and brings a performance gain of up to 4.75% for the Q&A task (comparable to domain-adaptive pre-training in other domains). The framework, the generated CyLLMs, and the data are publicly available for use in cybersecurity applications.
■Comment
Attending ICISSP 2025 in Porto and receiving the Best Student Paper Award are significant milestones of my study at JAIST. Although there were problems with connecting flights and presentation schedule, I successfully presented my paper and shared my work with cybersecurity researchers from around the world. Throughout the three days of the conference, I had the opportunity to communicate with other researchers and learn many things from them, which will help improve the quality of my future research.
I would like to thank my supervisors, Associate Professor Razvan Florin Beuran and Associate Professor Naoya Inoue, for their guidance regarding the research presented in this paper. I would also like to thank JAIST for providing the high-quality infrastructure (HPC system) that enabled this research.
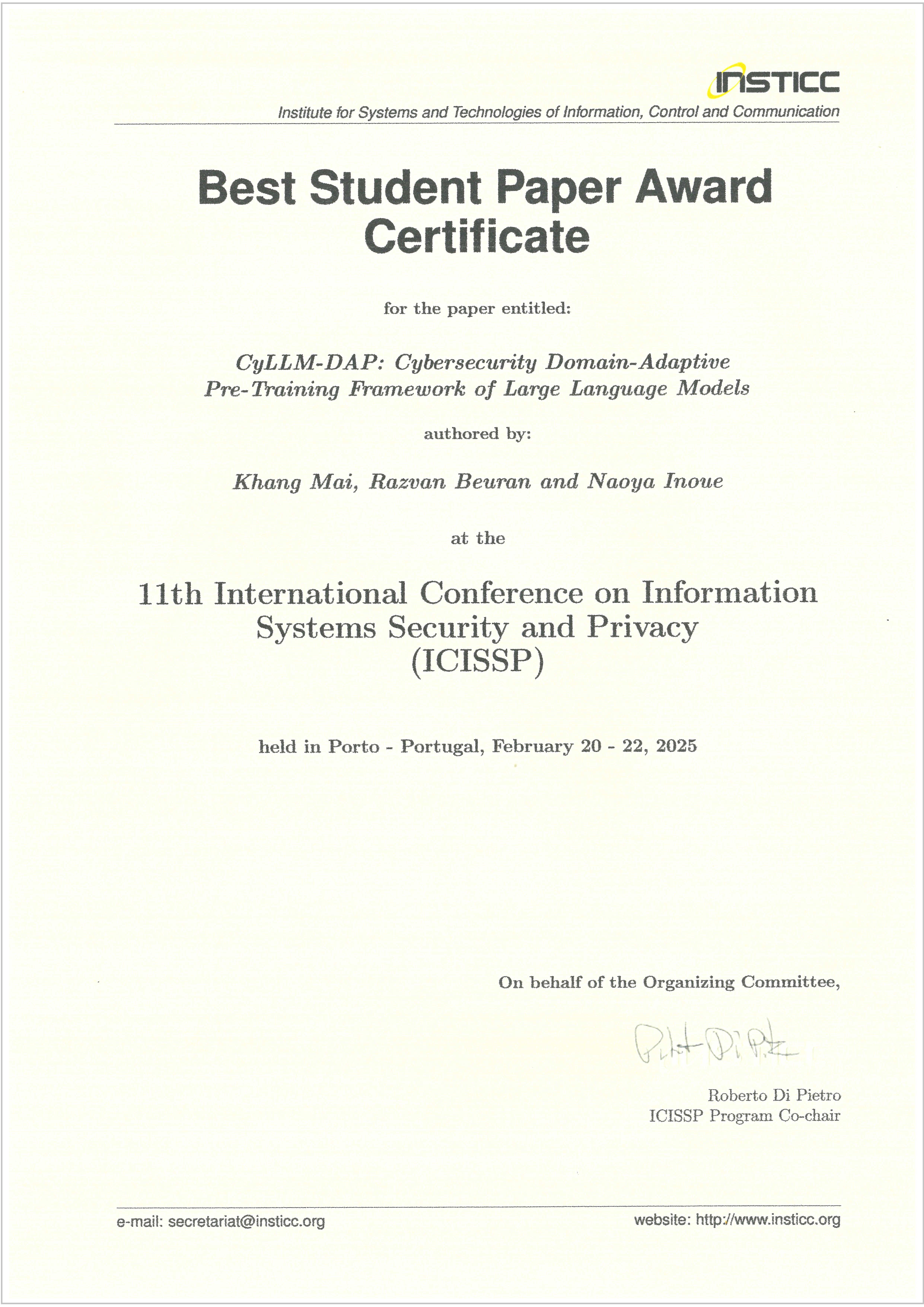
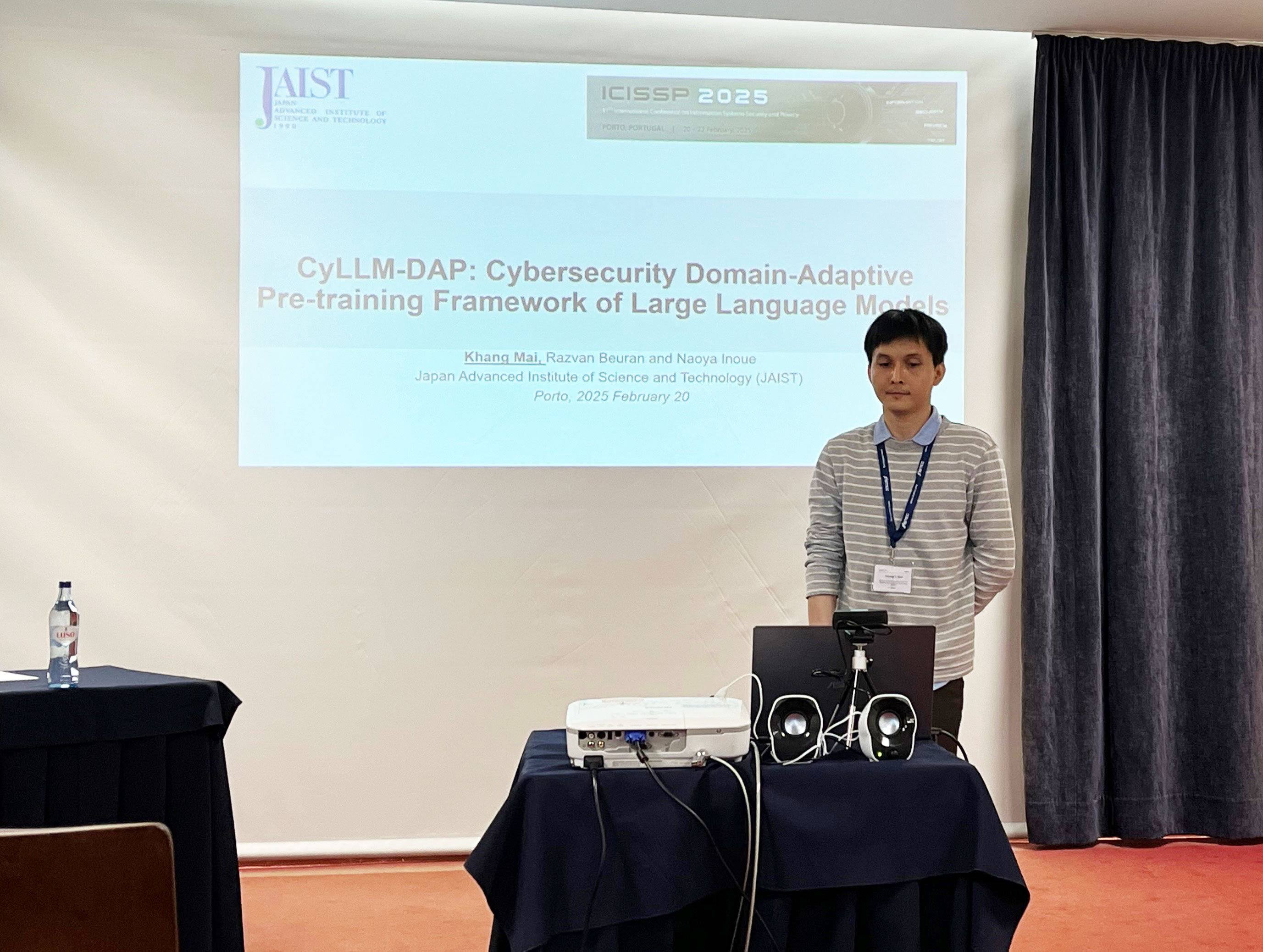
March 13, 2025